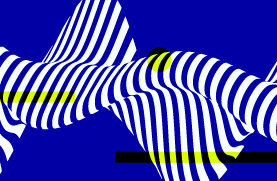
Resumen: Many events and policies (treatments), such as opening of businesses, building of hospitals, and sources of pollution, occur at specific spatial locations, with researchers interested in their effects on nearby individuals or businesses (outcome units). However, the existing treatment effects literature primarily considers treatments that could experimentally be assigned directly at the level of the outcome units, potentially with spillover effects. I approach the spatial treatment setting from a similar experimental perspective: What ideal experiment would we design to estimate the causal effects of spatial treatments? This perspective motivates a comparison between individuals near realized treatment locations and individuals near counterfactual (unrealized) candidate locations, which is distinct from current empirical practice. I derive standard errors based on this design-based perspective that are straightforward to compute irrespective of spatial correlations in outcomes. Furthermore, I propose machine learning methods to find counterfactual candidate locations and show how to apply the proposed methods on observational data. I study the causal effects of grocery stores on foot traffic to nearby businesses during COVID-19 shelter-in-place policies. I find a substantial positive effect at a very short distance. Correctly accounting for possible effect “interference” between grocery stores located close to one another is of first order importance when calculating standard errors in this application.
Acerca del expositor: Michael Pollmann is an Assistant Professor of Economics at Duke University.
Tiempo de exposición: 1 hora
Seminario virtual organizado por Cali, Cartagena y Medellín.